Course Overview
Our Analytics Engineering course is fully self-paced — students can expect to spend 3-4 hours a week working their way through lectures and exercises. We suggest spreading out the course over 10 weeks with a suggested timeline below.
On this page
Tools you’ll master
- The command line
- Git and GitHub
- dbt (data build tool)
- Cloud data warehouses
- Python
Note: We’ll be using BigQuery in the course, but most of what we learn will be relevant for users of Redshift and Snowflake.
Skills you’ll learn
- Testing patterns (in SQL and Python)
- Code collaboration via git
- Data modeling
- Advanced SQL patterns
- Debugging
- Data warehouse performance optimization
Curriculum
We previously taught this course over 10 weeks with the lessons grouped into the following modules. You may choose to follow along or choose your own adventure!
Week 1
Prework
Before the course, we’ll ask you to spend about 30 minutes getting your computer set up, and writing a SQL query, which we’ll use as the basis for later lessons. We encourage students to introduce themselves and meet everyone in our Slack group!
Week 2
Command line basics | Version control & Git
Week 1 covers a lot of ground — for some students most of this content will be familiar, but for others it may be mostly new! We (and the rest of your cohort) will be here to support you.
First, we’ll learn how to use the command line to navigate our computers — important groundwork for many of the tools we’ll learn in the course!
Then, we’ll cover all things about version control and Git! From creating repos, and making changes, all the way to handling merge conflicts.
We’ll also discuss SQL style and use our new git workflow as an opportunity to refactor a query.
Week 3
Transforming data with dbt
We’ll learn what dbt is, and build our first dbt project together complete with tests and documentation. Along the way, we’ll learn about DAGs, deployment environments, and running jobs in production.
Week 4
Data modeling
Data models, star schemas, denormalization, Kimball — what do they all mean, and how relevant are they anyway? We’ll talk you through some of these concepts, talk about the process of designing a data model as an analytics engineer. You’ll spend time building your own data models in your dbt project.
Week 5
Advanced SQL
This week will be all about SQL! We’ll look at some of the common patterns we see as analytics engineers and implement them in our dbt project — we’ll write the SQL to resolve user identities, perform a cohort analysis, fan out (or date spine) your data, and aggregate page views to web sessions.
Week 6
Data warehouses
This week will be a lot of theory and the study of how databases work fundamentally, and how data warehouses specifically are optimized. We’ll cover everything from row vs column store to the basics of distributed computing. By the end of this lesson, you’ll understand how to tune up a data warehouse and the theory behind it.
Weeks 7 & 8
Advanced dbt
This week we’ll dive into some of the more advanced features of dbt — writing Jinja, using packages, and some of the advanced materializations available.
Weeks 9 & 10
Python for analytics engineers
If you’re brand new to python, we’ll start off by introducing some of the basics of the language.
Then, we’ll learn how to write python like an engineer — we’ll cover running python outside of a notebook, setting up virtual environments, code style, tests, and modularity. By the end of these two weeks, you’ll have all the tools at your disposal to write your own scripts, command-line tools, and even consider contributing to an open-source package!
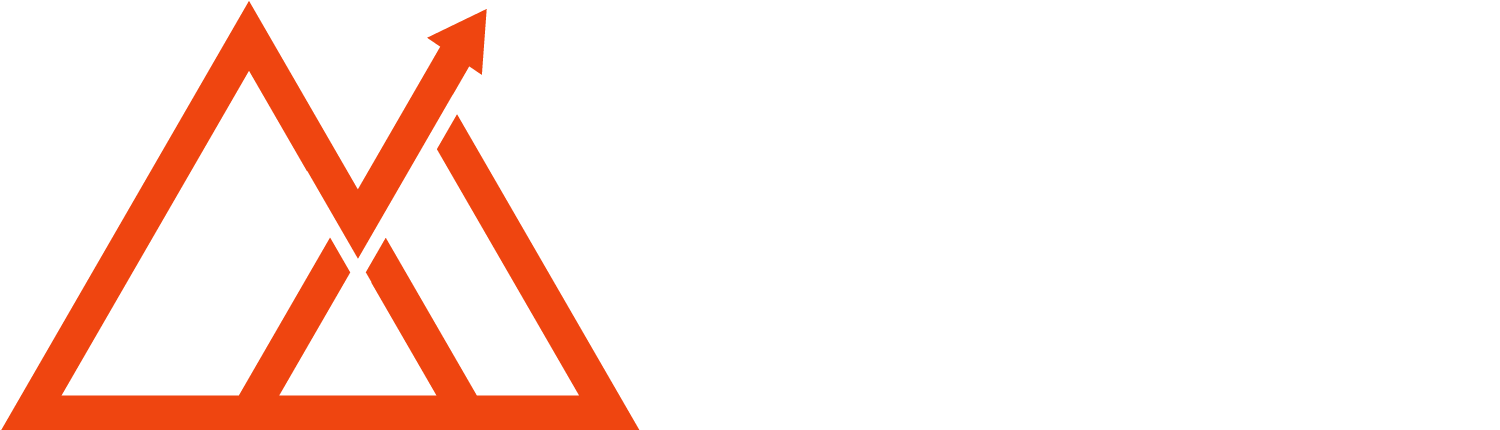
Contact Us
hello@analyticsengineers.club
© 2021 Analytics Engineers Club. All rights reserved.
Join the club
Join our mailing list to be the first to know when registrations open, or to keep an eye on our blog.